Transfer Learning for Battery Smarter State Estimation and Ageing Prognostics
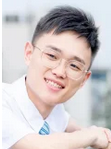
Special Issue Information
Dear Colleagues,
With the advent of sustainable and clean energy transitions, lithium-ion batteries have become one of the most important energy storage sources for many applications. Battery management is of utmost importance for the safe, efficient, and long-lasting operation of lithium-ion batteries. However, the frequently changing load and operating conditions, the different cell chemistries and formats, and the complicated degradation patterns pose challenges for traditional battery management.
The data-driven solutions that have emerged in recent years offer great opportunities to uncover the underlying data mapping within a battery system. In particular, transfer learning improves the performance of data-driven strategies by transferring existing knowledge from different but related domains, and if properly applied, would be a promising approach for smarter battery management. The states estimation and aging prognostics with transfer learning are promising to address the challenges of lacking accuracy and generalization for most data-driven methods.
Therefore, this special issue is focusing on the advanced transfer learning strategies, such as model -finetuning and domain adaptation, for smarter battery management.