Defocused image restoration method based on micro-nano scale
Yongjun Liu, Qiuyu Wu, Mingxin Zhang, Yi Wang
Article ID: 1335
Vol 4, Issue 2, 2021
VIEWS -
2810
(Abstract)
Abstract
An image adaptive noise reduction enhancement algorithm based on NSCT is proposed to perform image restoration preprocessing on the defocused image obtained under the microscope. Defocused images acquired under micro-nano scale optical microscopy, usually with inconspicuous details, edges and contours, affect the accuracy of subsequent observation tasks. Due to its multi-scale and multi-directionality, the NSCT transform has superior transform functions and can obtain more textures and edges of images. Combined with the characteristics of micro-nanoscale optical defocus images, the NSCT inverse transform is performed on all sub-bands to reconstruct the image. Finally, the experimental results of the standard 500 nm scale grid, conductive probe and triangular probe show that the proposed algorithm has a better image enhancement effect and significantly improves the quality of out-of-focus images.
Keywords
Micro-nano Scale; Defocused Image; NSCT; Image Restoration
References
Hu R. Scanning near-field optical microscopy: Instrumentation and applications [Master’s thesis]. Nanjing: Nanjing University; 2018. p. 91.
Lv M, Yu Z. Study on automatic focusing algorithm of optical microscope. China Measurement & Test 2018; 44(6): 11–16.
He Z. Research on depth recovery based on defocused images [Master’s thesis]. Harbin: Northeast Agricultural University; 2014. p. 62.
Zhu Z, Yin H, Chai Y, et al. A novel multi-modality image fusion method based on image decomposition and sparse representation. Information Sciences 2018; 432: 516–529.
Chen BH, Wu YL. An entropy-preserving histogram modification algorithm for image contrast enhancement. Proceedings of the 2017 IEEE International Conference on Applied System Innovation (ICASI); 2017; Sapporo. New York: IEEE; 2017. p. 1285–1288.
Zhang S. Research on enhancement algorithms of low illumination images and videos based on Retinex theory [Master’s thesis]. Nanjing: Nanjing University of Posts and Telecommunications; 2018. p. 76.
Gao Y, Liu G, Ma C. Dense hazy image enhancement based on generalized imaging model. 2018 IEEE 3rd International Conference on Image, Vision and Computing (ICIVC); Jun 27–Jun 29, 2018; Chongqing. New York: IEEE; 2018. p. 410–414.
Zhang D. Research on adaptive compressive sampling imaging based on wavelet transformation [Master’s thesis]. Chengdu: Southwest Jiaotong University; 2018. p. 69.
Liu Z, Xu T, Song Y, et al. Image fusion technology based on NSCT and robust principal component analysis model with similar information. Journal of Jilin University (Engineering and Technology Edition) 2018; (5): 1614–1620.
Liu L. Research on image enhancement algorithm based on NSCT [Master’s Thesis]. Urumqi: Xinjiang University; 2016.
Pal NS, Lal S, Shinghal K. A robust framework for visibility enhancement of foggy images. Engineering Science and Technology 2019; 22(1): 22–32.
Chen BH, Wu YL, Shi LF. A fast image contrast enhancement algorithm using entropy-preserving mapping prior. IEEE Transactions on Circuits and Systems for Video Technology 2017; 20(2): 212–232.
DOI:
https://doi.org/10.24294/can.v4i2.1335
Refbacks
- There are currently no refbacks.
Copyright (c) 2021 Yongjun Liu, Qiuyu Wu, Mingxin Zhang, Yi Wang

This work is licensed under a
Creative Commons Attribution-NonCommercial 4.0 International License.
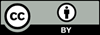
This site is licensed under a Creative Commons Attribution 4.0 International License.