Tumor Segmentation
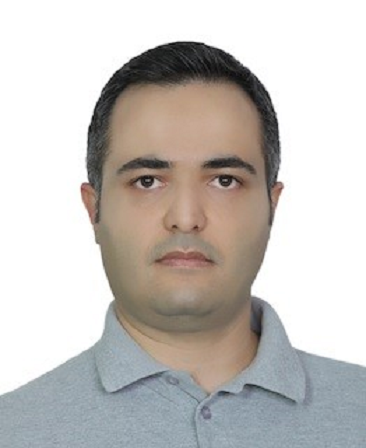
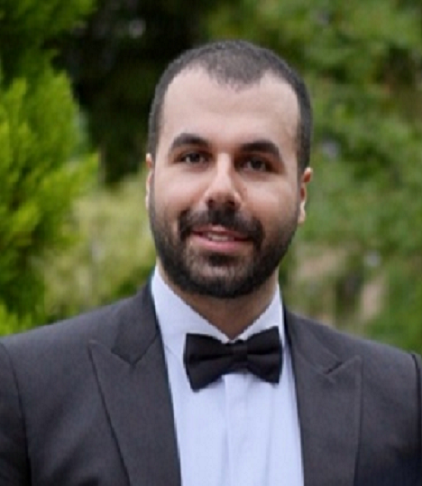
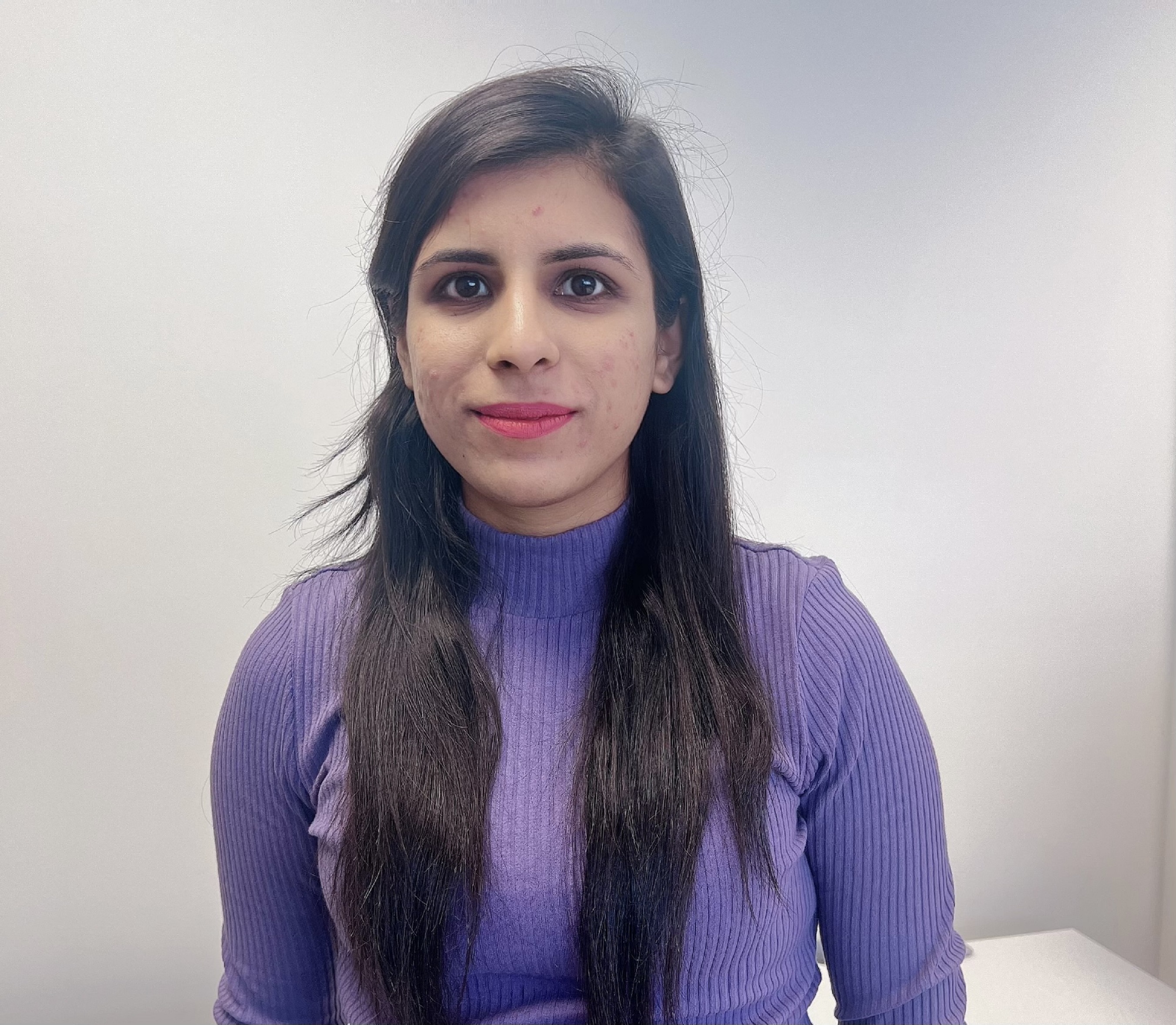
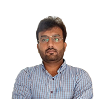
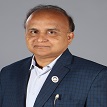
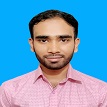
Section Collection Information
Tumor segmentation is an indispensable facet of medical image analysis, serving as the cornerstone for the accuracy of cancer diagnostics and the strategic planning of treatment regimens. In the era preceding the advent of advanced computational techniques, segmentation processes predominantly relied on manual intervention. This reliance was a source of extensive procedural delays and a lack of uniformity in diagnostic outcomes, thereby impeding the scalability and reliability of oncological assessments. Contemporary strides in deep learning, epitomized by Convolutional Neural Networks (CNNs) and nascent paradigms such as Transformer and Transformer-CNN hybrids, have revolutionized this task.
These advanced methodologies harness neural networks’ capacity to autonomously discern and distill features from medical imagery, encompassing modalities like MRI, CT, and PET scans. This automation facilitates enhanced precision and expediency in tumor delineation. In the contemporary landscape, convolutional architectures such as U-Net, Fully Convolutional Networks (FCN), and Mask R-CNN have emerged as paragons of innovation, undergirding the automated segmentation of tumors with remarkable success. These frameworks have been pivotal in delineating neoplastic tissues with high fidelity, demonstrating their versatility and effectiveness across a diverse array of cancerous pathologies. The scarcity of voluminous, annotated datasets and the imperative for algorithmic generalization across disparate imaging techniques and tumor typologies persist as significant hurdles. Research trajectories are converging on the development of algorithms with heightened robustness and adaptability, the amalgamation of multimodal data, and the exploration of innovative methodologies, including weakly supervised learning and domain adaptation.
The integration of these advanced computational models into clinical practice holds the promise of revolutionizing patient care. The deployment of these cutting-edge technologies is anticipated to streamline treatment planning and refine prognostic assessments significantly, thereby fostering the development of more tailored and efficacious cancer treatments. As the discipline progresses, the confluence of computational innovation and clinical acumen is expected to establish unprecedented standards in oncology that prioritize the patient’s individual needs and circumstances.