Advanced Techniques in Ultrasound Image Analysis
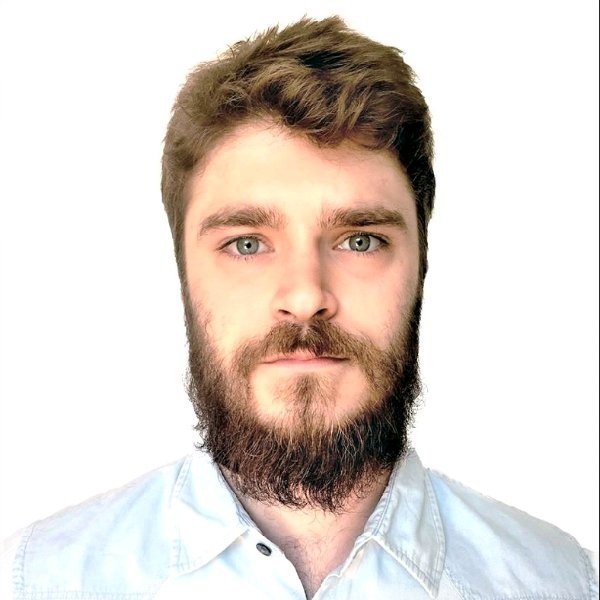
Section Collection Information
Dear colleagues,
Ultrasound imaging is a pivotal diagnostic tool in medicine, offering real-time visualization of internal body structures. Advanced techniques in ultrasound image analysis have significantly enhanced diagnostic accuracy, efficiency, and the scope of applications. These techniques leverage sophisticated algorithms and computational methods to improve image quality, automate feature extraction, and enable quantitative analysis.
Speckle Reduction and Image Enhancement: Speckle noise is a common challenge in ultrasound images, obscuring fine details and reducing contrast. Advanced speckle reduction techniques, such as adaptive filtering, wavelet transform, and non-local means algorithms, have been developed to enhance image clarity and detail. These methods improve the visibility of structures and lesions, aiding in more accurate diagnosis.
3D and 4D Ultrasound Imaging: The advent of 3D and 4D ultrasound imaging has revolutionized the way clinicians view and interpret ultrasound data. These technologies provide volumetric imaging capabilities, offering comprehensive views of anatomical structures and dynamic processes. Advanced algorithms for volume rendering, multi-planar reformatting, and automated segmentation facilitate detailed analysis and improve the understanding of complex anatomical relationships.
Elastography: Elastography is a technique that measures the elasticity or stiffness of tissues, providing valuable information about pathological changes. Advanced elastography methods, such as shear wave elastography and strain elastography, have been integrated into ultrasound systems. These techniques are particularly useful in the detection and characterization of tumors, liver diseases, and vascular abnormalities.
Machine Learning and AI: Machine learning and artificial intelligence (AI) have been increasingly applied to ultrasound image analysis. These technologies enable the automated detection and classification of diseases, reducing the reliance on manual interpretation. Deep learning algorithms, trained on large datasets, can identify patterns and features that may be subtle or overlooked by human observers. AI-driven tools also support quantification, predictive modeling, and personalized medicine by analyzing ultrasound data in conjunction with patient-specific information.
The incorporation of texture analysis and quantitative ultrasound (QUS) adds another dimension to ultrasound image analysis, enabling the characterization of tissue structures at a microstructural level. Texture analysis involves the extraction of features from ultrasound images that reflect the distribution of pixel intensities, revealing patterns that are not discernible to the human eye. These patterns can indicate the presence of disease or the progression of a condition. Quantitative ultrasound goes further by measuring acoustic properties of tissues, such as attenuation and backscatter coefficients, which can be correlated with tissue composition and pathology. QUS has shown promise in various applications, including the assessment of liver fibrosis and the differentiation of benign from malignant breast lesions, offering a non-invasive and cost-effective diagnostic tool.